The Promise of Wearables
Wearables offer the possibility of continual monitoring for Covid-19 and changing the game on catching infectious people
Welcome to Plugging the Gap (my email newsletter about Covid-19 and its economics). In case you don’t know me, I’m an economist and professor at the University of Toronto. I have written lots of books including, most recently, on Covid-19. You can follow me on twitter (@joshgans) or subscribe to this email newsletter here.
Note: this week I am switching from a daily newsletter to just Monday, Wednesday and Friday.
Most discussion of closing the Pandemic Information Gap has focused on tests and making them cheap, effective, and immediate. But wearables, that monitor peoples’ health continuously, offer a method of getting ahead of what might be achieved by a testing cycle in a more convenient way. Today’s post looks at a new study from Fitbit that suggests that potential may be realised.
In a pre-print, that has not yet been peer-reviewed, researchers from Fitbit reported on results of a study of 100,000 Fitbit users in the US and Canada of which 1,181 ended up testing positive for Covid-19. The study found that a combination of self-reported symptoms, as well as device, measured respiration and heart rate information while asleep could predict the on-set of Covid-19 a day or two before it would be symptomatic. It could also predict hospitalisations.
The study isn’t perfect but it is interesting. Let’s start with the device-based information which one would guess is more reliable and consistent. Here is the main finding in a chart:
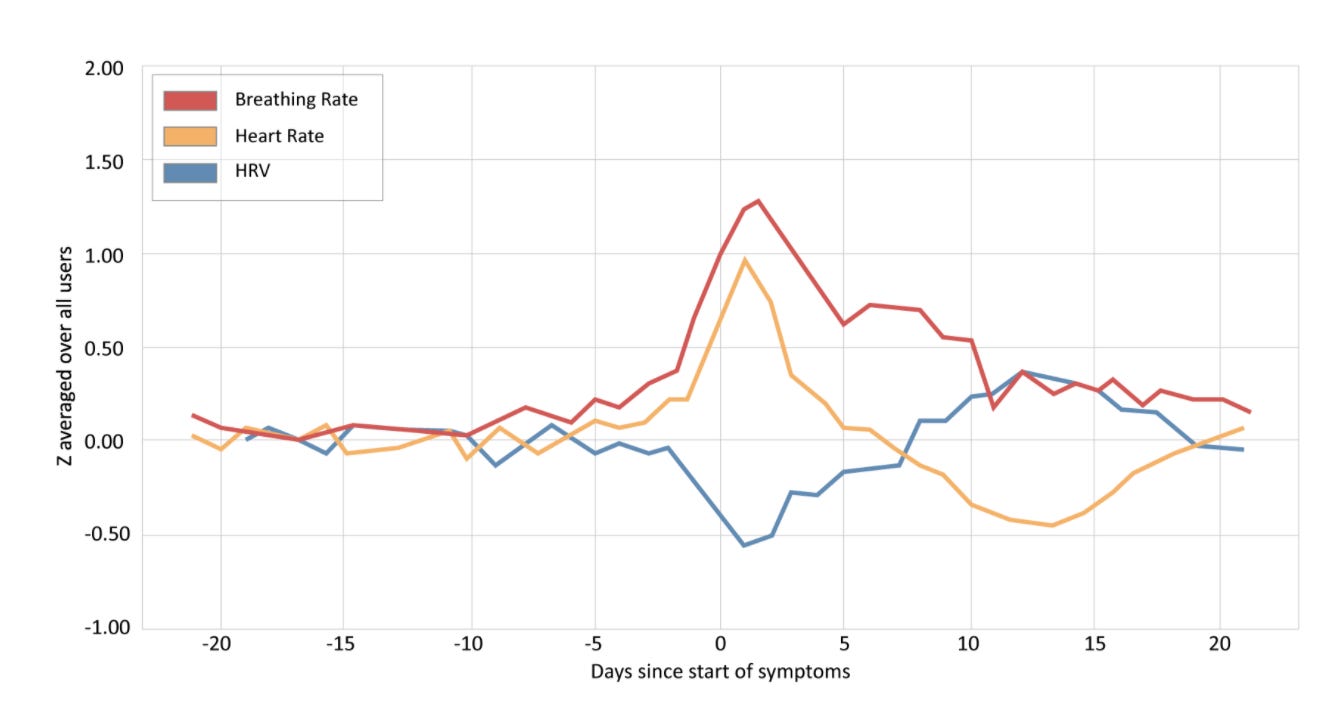
What this shows is that just before Covid-19 symptoms are observed (i.e., Day 0), the breathing and heart rate rise while the variability in the heart rate falls. That seemed pretty interesting and caused me to look at my Apple watch data to see if things had changed at some point for myself. (The answer is that yes they did. Resting heart rate and HRV went up at the beginning of March just after I had returned from a trip to the US. Dum da dah! I recall that I was definitely psycho-symptomatic then.)
Combining this data with the self-reported symptom data, the researchers then used machine learning to develop a prediction of Covid-19 for any given day. The resulting classifier can detect (depending on the choice of specificity) between 21 and 29% of cases one day before symptoms begin. That is a pretty good result given that, without a test that happened to be conducted that day, we can’t predict anything.
The reason why these results depend on specificity is that in designing a predictive algorithm, the researchers face a trade-off between sensitivity (the number of false negatives) and specificity (the number of false positives). [For more on sensitivity and specificity, see my earlier post]. That trade-off can be shown for this algorithm:
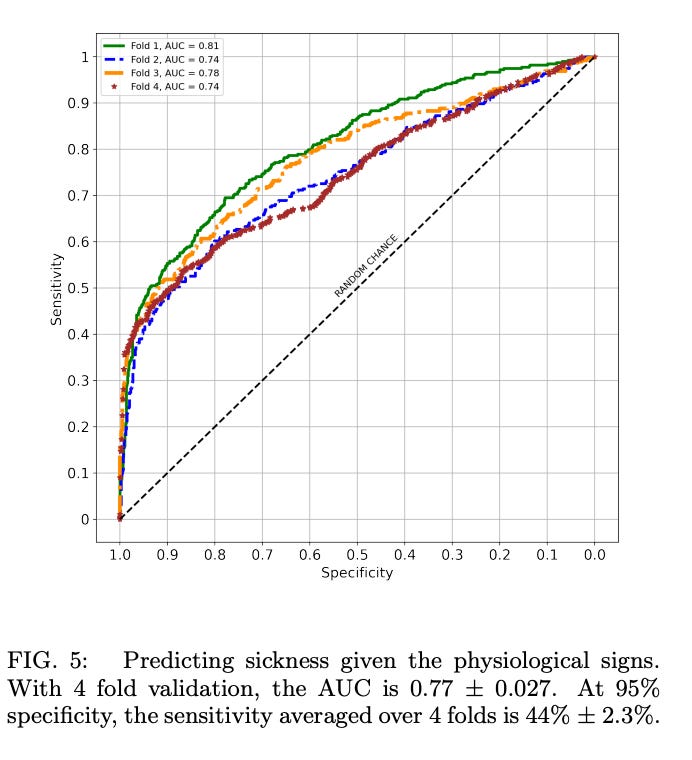
A terrific algorithm would have a curve the shot up vertically quickly before pivoting to become horizontal at a sensitivity close to 1. This one isn’t there but it is typical of many predictive algorithms. The key point is that if the algorithm has a 95% specificity (akin to what many PCR tests for the coronavirus have), then the sensitivity is 44%. This means that if you are positive for the coronavirus and have been monitoring using a Fitbit or other device, Fitbit’s algorithm will pick that up 44% of the time. Compared with the rate for an asymptomatic person with no test, that is quite an advance.
The study also found some other information regarding symptoms:
In addition, our study found that shortness of breath and vomiting are the symptoms most likely to predict that someone with COVID-19 will need to be hospitalized, while sore throat and stomachache were the symptoms least likely to predict the need for hospitalization.
We’re also seeing that the most common symptom reported by individuals with COVID-19 was fatigue, which was present in 72 percent of participants reporting having COVID-19. This was followed by headache (65 percent), body ache (63 percent), decrease in taste and smell (60 percent), and cough (59 percent). Of note, fever was present in just 55 percent of people reporting COVID-19, an indicator that temperature screening alone may not be enough to understand who might be infected.
Mild cases (those who recovered at home on their own) show a median duration of 8 days, while moderate cases (those who recovered at home with help from others) last about a week longer, with a median duration of 15 days. For severe cases (patients who end up requiring hospitalization), the median duration of illness was approximately 24 days. But this duration had a large spread, with several cases lasting longer than two months.
So, if this finding holds, this actually means you can worry less if you have a sore throat and a stomachache than you might otherwise which is good news (I think) for psycho-symptomatics.
There is a lot we don’t know. How representative was this sample? How annoying would alerts that you might have Covid-19 be? And we have to recognise that this has come from Fitbit, an interested party, but then again they also have a big interest in ensuring that this works can be validated.
Nonetheless, with regard to testing, no matter how cheap and available those tests are, they have to be taken. The same is true of temperature checks. With wearables, the monitoring is continuous and the human factor can be removed. That provides a key opportunity for health management, especially for infectious diseases. The World Economic Forum is actively pursuing wearables to help businesses deal with Covid-19.
Fitbit just announced a sensor-packed Fitbit Sense to gather even more data for this purpose. At over $300 a pop, however, this won’t be the solution for Covid-19 but may be a solution for Covid-29.
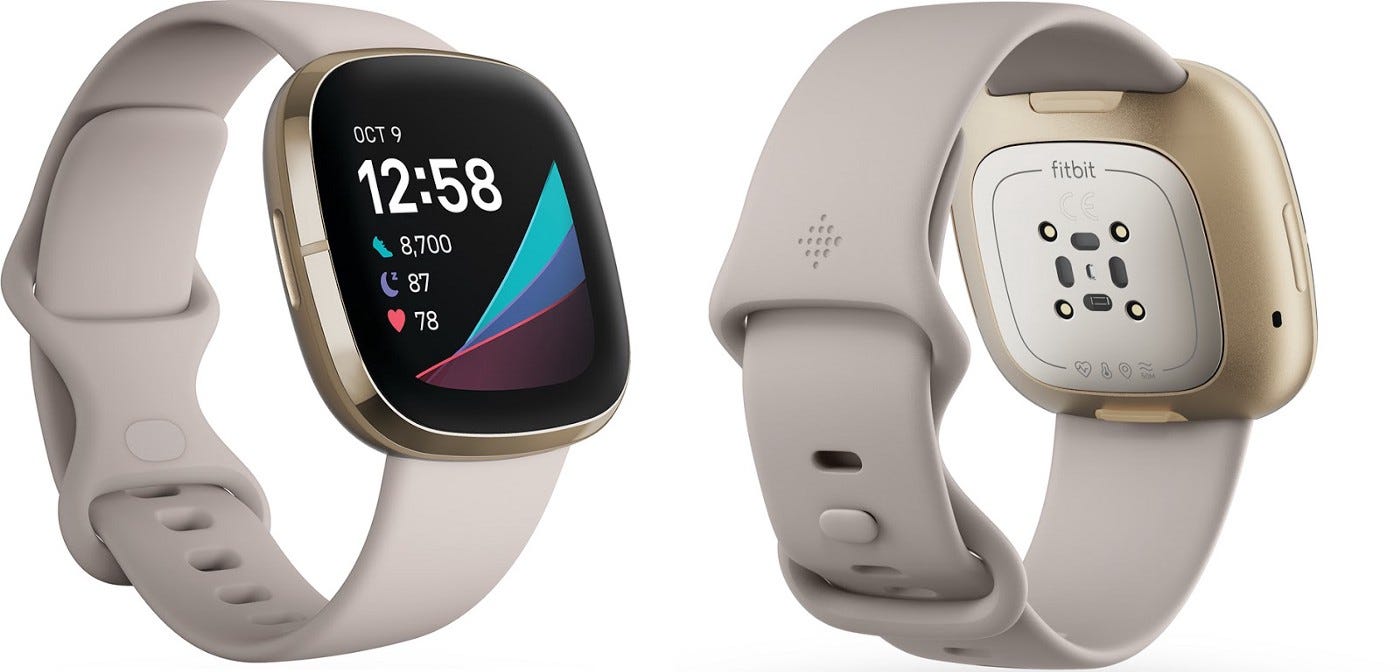