Test Week: Finding the Right Fit
All tests involve some errors but the type of error matters in finding the test that fits the decision being made
Welcome to Plugging the Gap (my email newsletter mainly about Covid-19 and its economics). My goal is for several posts a week explaining economic research and the economic approach to understanding the pandemic. (In case you don’t know me, I’m an economist and professor at the University of Toronto. I have written lots of books including most recently on Covid-19. You can follow me on twitter (@joshgans) or subscribe to this email newsletter here).
This week is ‘Test Week’ for this newsletter. Yesterday’s post looked at how tests can solve the pandemic information gap and reduce the need for costly interventions such as social distancing, PPE and cleaning. Today, we look at the tests themselves. Tests for whether someone has the coronavirus have different efficacy. Some are more precise than others while, for most, they involve distinct mixes of false positives and false negatives. Depending on the decision you are using the test for, your tolerance for these errors can be very different. In particular, for diagnosis decisions, you want there to be few false positives and for clearance, you want the tests to have few false negatives. Critically, approval processes for tests are based solely on diagnosis which leads to evaluations that are misleading for other contexts.
When radar was invented in World War II, there was an immediate issue. If you saw an object appear on a radar screen, how did you know if it was really, say, an enemy plane or some noise, say, caused by a flock of birds? The further the target is away from the radar itself, the more noise is present. The decision the operator faced was whether to sound an alarm or not. The errors that were possible were a false alarm (sounding the alarm when there was no real target) or a miss (not sounding the alarm when there was a real danger). It won’t surprise you that, in a military situation, the costs of a miss were greater than the costs of a false alarm and so radar operators were told to err on the side of sounding an alarm.
It would be a great world if we had perfect tests for the presence of the coronavirus. Then we would know if someone was infected or not and would be able to take action accordingly. But when there are errors, then we have to worry not only about how often those errors arise but also what the relative costs of different errors are. This is made all the more important because, in the design of any test, we make choices regarding the mix of errors that might arise presuming that our actions resulting from a test will then be straightforward: that is, if someone is positive, then isolate/treat and if they are negative, then relax. In other words, we ask of our tests a straight up or down result where, in fact, there is more information there which we end up throwing away.
How the standard PCR tests work
The PCR or “reverse-transcription polymerase chain reaction” test is quite an amazing thing. It was invented in 1983 and made the Human Genome Project possible. Here is a nice description from an article in The Atlantic last week:
It works, in essence, like a zoom-and-enhance feature on a computer: Using a specific mix of chemicals, called “reagents,” and a special machine, called a “thermal cycler,” the PCR process duplicates a certain strand of genetic material hundreds of millions of times.
When used to test for COVID-19, the PCR technique looks for a specific sequence of nucleotides that is unique to the coronavirus, a snippet of RNA that exists nowhere else. Whenever the PCR machine—as designed and sold, for instance, by the multinational firm Roche—encounters that strand, it makes a copy of both that sequence and a fluorescent dye. If, after multiplying both the strand and the dye hundreds of millions of times, the Roche machine detects a certain amount of the dye, its software interprets the specimen as a positive. To have a “confirmed case of COVID-19” is to have a PCR machine detect the dye in a sample and report it to a technician. Tested time and time again, the PCR technique performs stunningly well: The best-in-class PCR tests can reliably detect, in just a few hours, as few as 100 copies of viral RNA in a milliliter of spit or snot.
This process is important because it provides a measure of the viral load. To find the needle in the haystack that is SARS-CoV2 RNA, it takes a strand and replicates it in a cycle. After each cycle, the machine looks for the required amount of dye that would indicate the presence of some amount of the RNA. If a sample has a high viral load, then that point might be reached in 5 cycles. If it is a low viral load, it can take more than 35 cycles. As a cycle is part of exponential growth, a Ct (or cycle count) score of 5 is many orders of magnitude lower than a Ct of 35. The number of cycles taken is, therefore, a measure of the quantity of the virus that is present. (If you think that this is a lot of technical detail for an economics blog, you are right but it turns out to be important). Interestingly, this means that a negative test is passing some number of cycles without triggering enough RNA detected. How many cycles? 40 seems to be the number. What is that threshold? Those are all choices that are part of the process and because this process is essentially an optimal stopping rule problem, the more you look, the longer the process takes and you can never by 100% sure the virus isn’t present in small amounts. In other words, this, like many other tests, is a statistical process.
Given this rap, it is not surprising that it is the standard by which the CDC measures all tests and why the majority of tests used thus far for the presence of Covid-19 have been PCR tests. But nothing good comes for free. These tests are expensive — between $50 and $150 a person — and they rely on machines and reagents to work which, it will not surprise you, have run into their own supply chain limitations during the pandemic.
Even with all of that, it is not perfect. First of all, you can have the coronavirus but the test result isn’t positive. This is especially the case in the first couple of days after the virus enters your system and as it replicates throughout your body it hasn’t shown up in your nasal passages yet. By Day 3, however, the PCR test can pick up the genetic remnants in your nasal passages or even saliva. But, assuming you develop symptoms at all, these won’t show up for a couple of days. So between Days 3 and 5, you are infectious or contagious but won’t know it unless you happen to have had a test during that time. And another thing — which we will talk about in detail tomorrow — is that even if you have a test of Day 3, it may take a day or two to get the results. So there is almost no hope of using a PCR test to catch people early and isolate them. That said, if someone was asymptomatic, that would not be the end of the story as there may be more time to pick someone up and do some good. But, given the cost, asymptomatic people are not encouraged to get tests and are not given priority for speedy results. Here is the problem with a gold standard. It is of high quality but it also has costs which cause you to economise on things.
Problems don’t stop after the first week of the virus. We are learning that after about 14 days, the coronavirus has done its damage in causing Covid-19. This is actually great news as the virus that is in your body is dead and so it can no longer do damage to others. But the problem is that the PCR test is a test to detect the virus and not how alive it is. In other words, you would still test positive. So you are infected with the virus but are not infectious or contagious. So if you are looking to the test to inform decisions on isolation or finding contacts, it is not even wrong. It is uninformative.
This video has a nice explanation of these issues that also goes on to cover some other issues I raise in what follows.
Sensitivity versus Specificity
For a medical diagnosis, the efficacy of tests is characterised by the concepts of sensitivity and specificity. The sensitivity of a test is the probability that someone, who does have Covid-19, tests positive for Covid-19. You would like that probability to be close to 1. The specificity of a test is the probability that someone, who does not have Covid-19, tests negative for Covid-19. You would also like this probability to be close to 1. The issue is that there is another choice variable inherent in a test with two outcomes (i.e., positive or negative): what is the threshold by which the outcome of a test is defined as positive if that threshold is exceeded and negative if it is not? Change the threshold and you potentially alter the sensitivity and specificity of a test — in opposite directions.
Before going into this, it might be useful to calibrate the terminology here. Recall that statistical inference of testing came out of signal detection theory for radars. There you were worried about false alarms (thinking it was a hostile plane when it wasn’t) and misses (failing to detect a hostile plane). In 1947, Jacob Yerushalmy realised that this same dilemma arose in medical diagnosis using X-rays. He wrote:
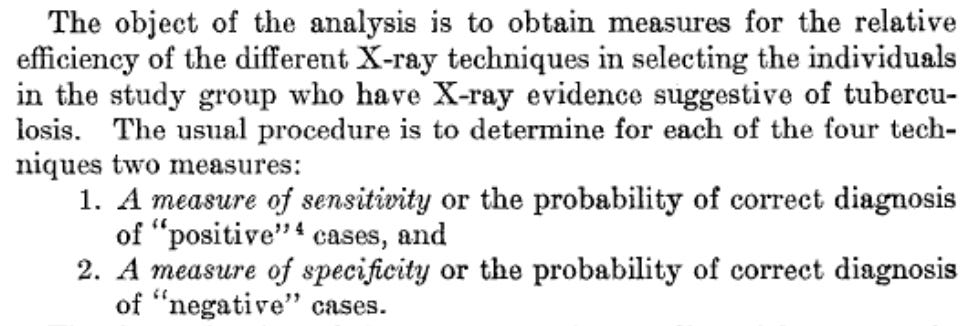
He didn’t explain why he chose those words but they stuck for medicine. In other situations, when evaluating tests or predictions people tend to focus on errors. So whereas sensitivity is the number of true positives divided by the number of positives (or the number of true positives plus the number of false negatives), others measure the number of false negatives as something that you would like to have less of. Similarly, whereas specificity is the number of true negatives divided by the number of negatives, others measure the number of false positives as something to be minimised. They are the same concepts just placed in a (ahem) positive or negative light.
Here is where I think the term sensitivity came from. When you undertake medical diagnosis, the key thing is something akin to resolution. How clear is your X-ray image? How easy is it to find an RNA remnant in a sample? A really sensitive test is one which can detect small amounts of something; that is, it has a higher resolution. In other words, the positive result is triggered even when the thing is hardly there. So it is a very sensitive instrument. By contrast, for specificity, you will record something as negative only when you have really, really searched for the thing and have given up. In other words, you try not to leave any stone unturned. There is a sense that for each, more effort can really improve outcomes but, in addition, if you have a limited amount of effort or time, you can’t have both of these things and so there is a trade-off.
Now that I am done with that historical interlude, let’s get back to PCR tests. PCR tests are favoured because they have sensitivity and specificity that both lie above certain thresholds. In particular, for sensitivity, they have been measured at 71 to 98% (akin to false-negative rates of 2-29%). If some of those numbers seem high, it is because those tests involve two steps the last of which is using the machine to find the RNA in the sample. The first step is to use a nasal swab to collect the sample and, if you have seen how far up the nose they go hunting for this, it won’t surprise you that there may be variation in how well that hunt goes. Done well, the sensitivity could be quite high.
For specificity, a commonly used number for PCR tests is 95% — in other words, there are few false positives. This article thinks specificity is more like 99.5%. In other words, if a PCR test finds you don’t have the virus — at least insofar as RNA remnants are concerned — then you really don’t have it. But we do have to be careful here. As I mentioned earlier, the test finds RNA remnants dead or alive. And over the course of even symptoms, the time a virus is dead can outstrip the time it is alive.
This is very important because the PCR test is used as a benchmark (or ‘gold standard’) by which all other tests are assessed. In other words, when they examine the efficacy of some other test for Covid-19, at the time same, they take a PCR test and examine the outcomes. If that test disagrees with the PCR test, that is how they calculate the sensitivity and specificity of that test. So if it so happened that the PCR test was wrong and the other test was right, the other test would be scored less! Suffice it to say, that is quite the game they have got doing there. To me, it kind of seems like those tests of voting eligibility in the Jim Crow era when you had to guess the number of jelly beans in a jar. The correct response is: by what standard?
It actually gets kind of worse. You may wonder: by what standard did they calculate the sensitivity and specificity of PCR tests? The answer is, by conducting other PCR tests! I don’t know where to start on that so I won’t.
We see then that PCR tests are considered to have high specificity (low false positives) and less high sensitivity (more false negatives). From a diagnosis perspective, this might make some sense. You don’t want to use up a hospital bed or treat someone who doesn’t have Covid-19 or instead has the flu. That is where you can end up “doing harm” and doctors have a motto against that. But let’s look at that from a clearance perspective; that is, a decision where you want to declare that someone is safe for close interaction with others. Using a helpful calculator from this article in the BMJ, we can calculate various outcomes if there is an 80% sensitivity and 99% specificity (as some of the better PCR tests have).
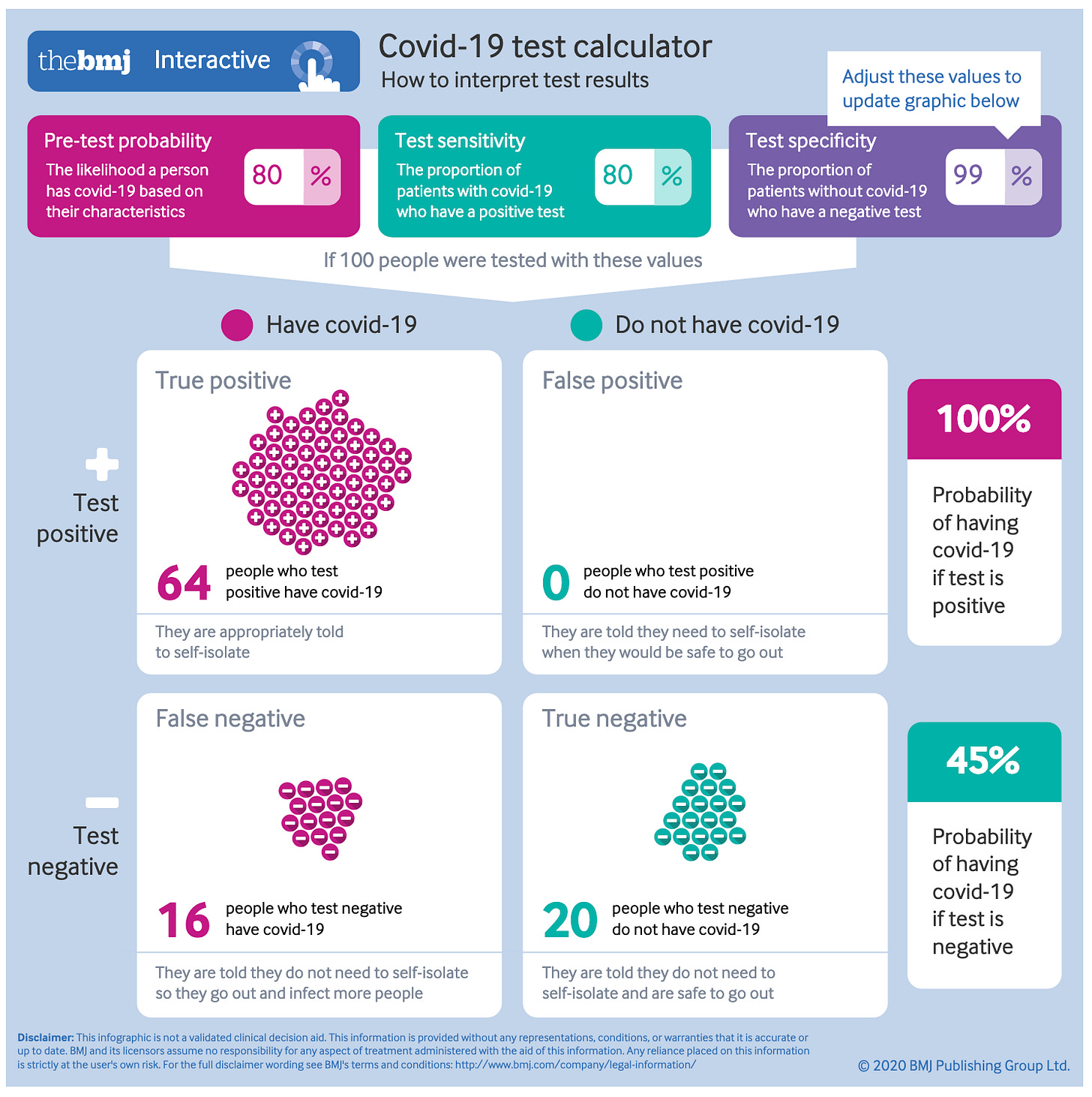
This first outcome assumes that you have a patient that you are pretty sure might have Covid-19 (a pre-test probability of 80%). In this case, the test does very well in confirming that. You only miss 16% of people you should be treating and the chances are, if they remain sick, you’ll test them again and pick them up then.
This second outcome is based on testing a random member of the population when the prevalence of the disease is 3% (which, as was discussed yesterday, has an impact on how you translate test results into the probability that someone is infected or not).
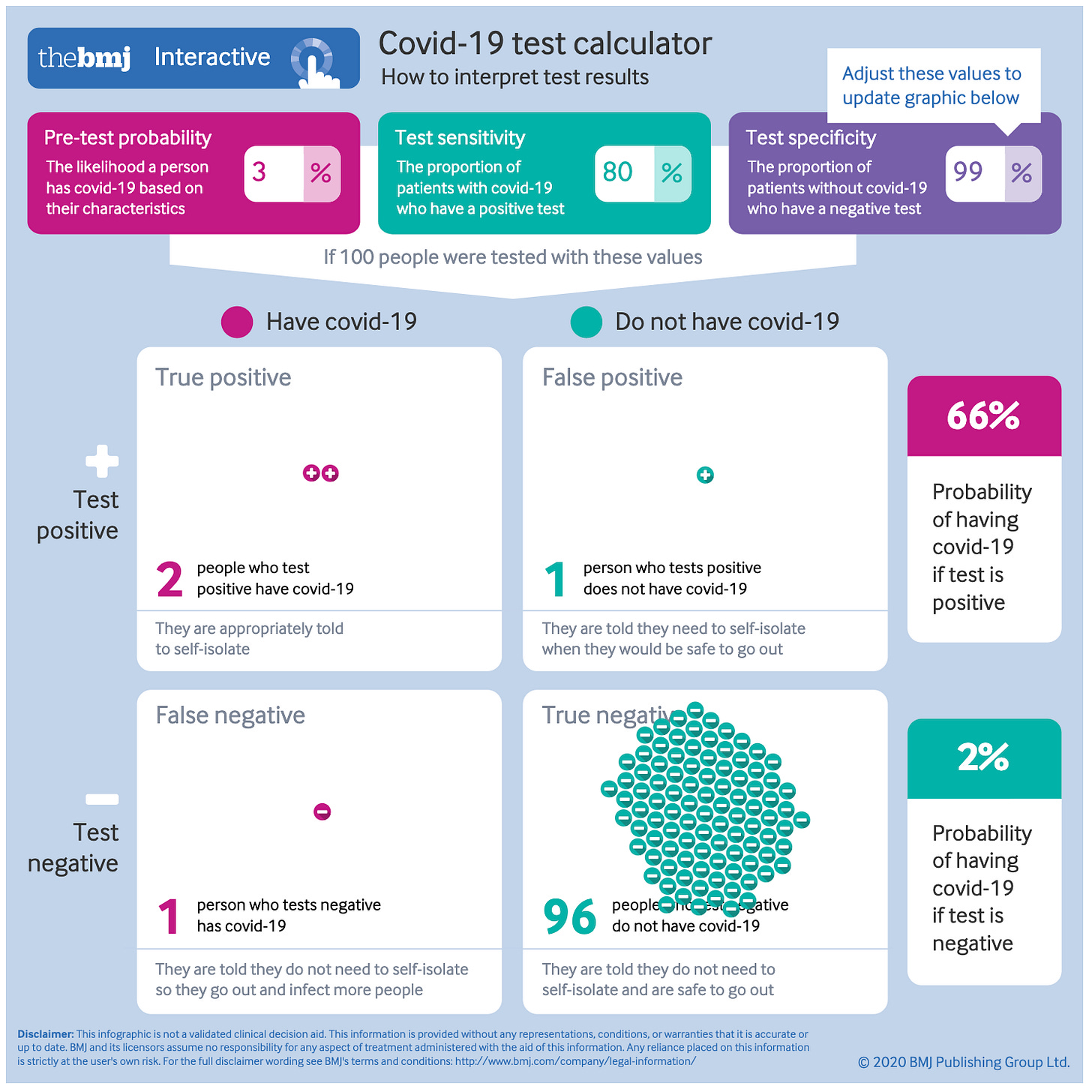
Most people test negative but of the people who test positive, one-third of them do not have Covid-19 but you also let one-third of the people who did have Covid-19 go.
If instead, you have a test with higher sensitivity but lower specificity, you would end up classifying more people as having Covid-19 but fewer people would be let back out with Covid-19 to infect others. When you are choosing outcomes for clearance — that is, who to allow to interact with others — the costs of someone being positive when they are not is just the cost of excluding them. Arguably that is a lower cost than misdiagnosis for treatment and so you are led to favour a different mix of error rates for your test.
To see what this looks like, say, for a test to allow you to work in a hospital, here is the calculation for a test with a 90% sensitivity but 80% sensitivity.
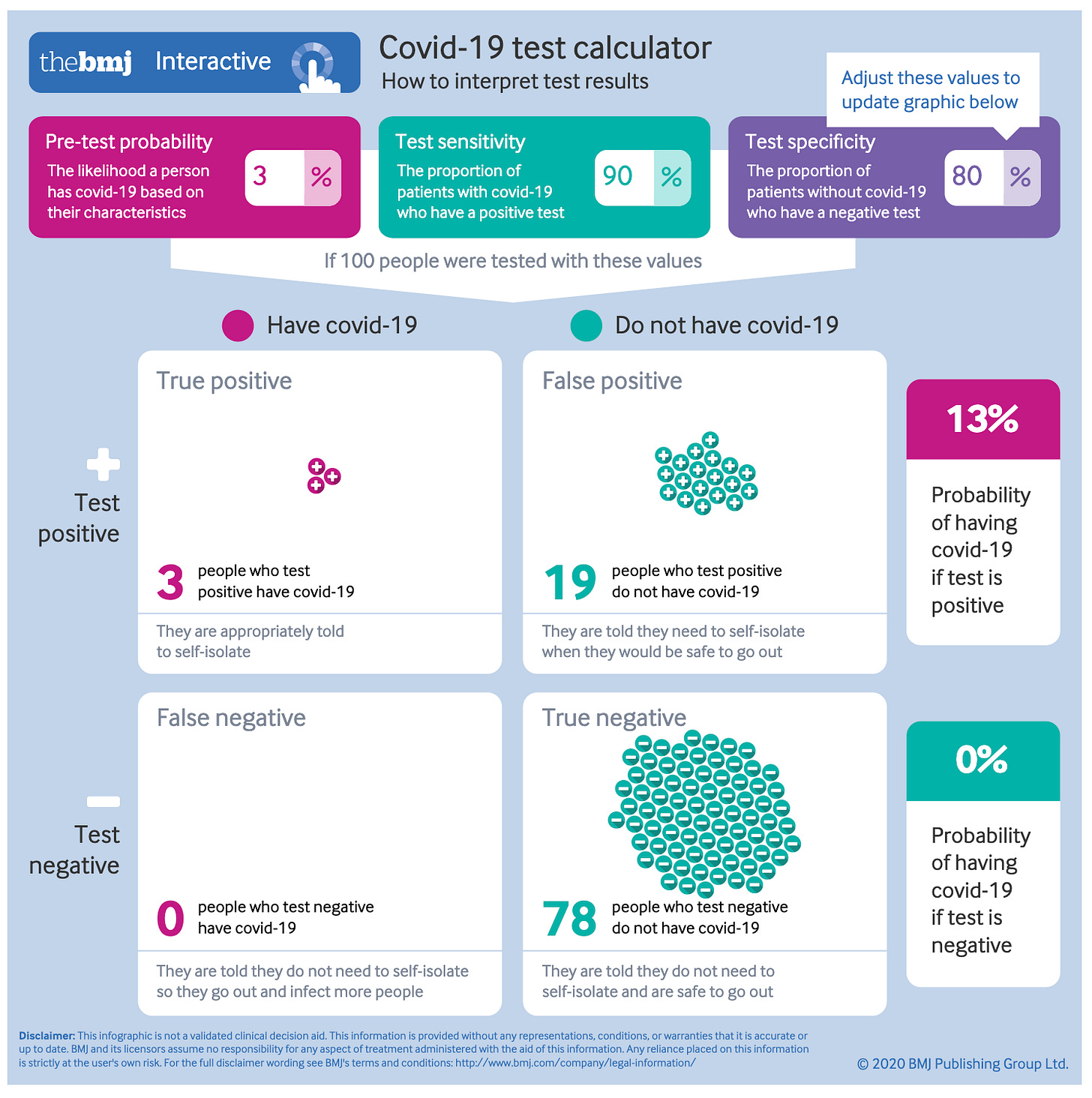
Notice that there are no false negatives and 78% of the people are cleared in this process.
This is relevant because a PCR test has thresholds that are chosen and hence, it is possible to choose a different threshold and change the sensitivity and specificity of a test. This is well known as explained here:
However, the problem with a Ct-based diagnosis is that there is no absolute or constant Ct cut-off value, and Ct cut-off values are different for each diagnostic reagent even for the same gene. For example, although there are differences according to diagnostic reagents, a sample is usually judged positive for COVID-19 based on a Ct value of 35. Although the Ct value in a rRT-PCR test is relatively accurate, error of 1~2 cycles are not uncommon in a Ct value depending on various factors, including the skill of the examiner. Therefore, when there is ambiguity in the Ct value, such as 34~36, the result may be interpreted as false negative or false positive depending on the Ct cut-off value.
The key phrase is highlighted. What you should know is that level of Ct is a very low one meaning that the PCR threshold is set so that a positive result can be returned even if there is very little of the coronavirus RNA remnants present in the solution. In other words, it is very specific. To come back negative, it really has to not find anything.
We have already noted that such a standard can cause the PCR test to pick up dead as well as live RNA remnants. But the critical thing is that when RNA remnants are live, that is when the coronavirus is making copies of itself that spread throughout the body and beyond. So if your primary interest was when the virus itself was alive in the body, as you would be if you are interested in whether the patient is contagious, you would choose a different threshold for a test to be negative — one where it is negative even if there are traces of RNA that are found but not many of them.
Moreover, if you are going to use the PCR test to judge the efficacy of other tests that may be used for a purpose other than diagnosis, it makes no sense to use a PCR test calibrated for the sensitivity and specificity you would want for diagnosis rather than that other purpose. But that is exactly how the sensitivity and specificity of other tests are calculated. No small wonder that non-PCR tests have hardly been approved for use anywhere.
The Testing Context
I am going to unpack this because it turns out to be really important for how tests are evaluated and scored. PCR tests are diagnostic tests and so are scored on sensitivity and specificity against a benchmark of some kind. But then when other tests for the presence of SARS-CoV2 are developed for different purposes, they are still scored on the same metrics.
Here is the problem with that. One set of tests that is emerging for the purpose of mitigation (that is, reducing the prevalence of the virus in the population by testing and then isolating) are antigen tests. I’ll spare you the details but these tests don’t look for genetic remnants but instead for chemicals that are highly present in the SARS-CoV2 virus. These tests have, however, been scored as far less sensitive than PCR tests — of the order of sensitivities of 50 or 60%. Hence, they have not seen as having a hope of being approved by the FDA who approves tests based on diagnostic use only.
The following diagram shows, however, that as a test for infectiousness rather than infection, these antigen tests may actually be highly sensitive for the mitigation purpose. The diagram shows the viral load from the point of infection. As noted earlier, the viral load doesn’t become high enough for someone to be infectious until about Day 3. It then remains high for up to a week before dropping down again including past a point where the virus that is present is not living. So what you want to do when testing for infectiousness is to have a test with a threshold that captures as much of the pink shaded area as possible. The problem is that the diagnostic benchmark for sensitivity compares the range of times when the PCR test comes back positive with the range of times (roughly) where the antigen test comes back positive. You can see why the antigen tests don’t score well because we are asking it to come up with a positive result at times when a positive result is not informative for its purpose.
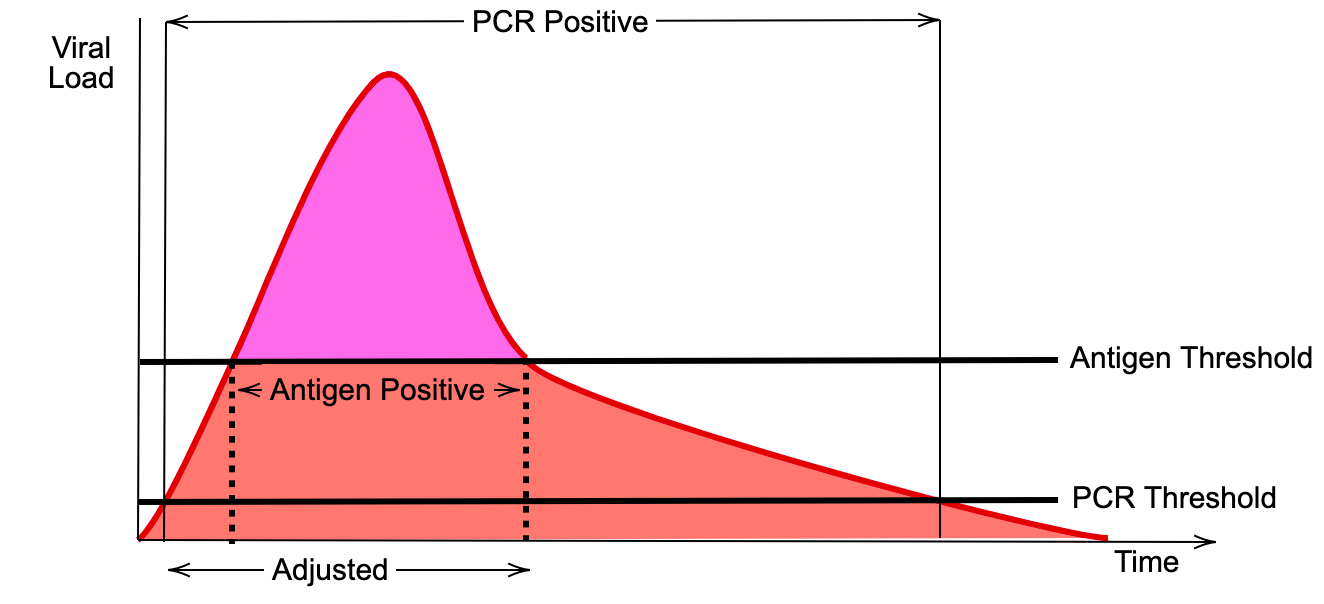
Instead, you want the benchmark to be the PCR test applied to the times when it is useful to isolate the patient from others. This is the ‘adjusted’ range in the diagram. You would compare the antigen test positive range to this. In other words, what the antigen potentially misses is that time close to the start of the infection whereby you infectiousness potential. So it is less sensitive. In sum, the probability that a positive antigen test implies that you are infected may be 50-60% but the probability that it implies that you are infectious may be 90% or above. (And this is not even taking into account the fact that if antigen tests are cheap, fast and easy you can apply them more often; something I will talk about more tomorrow).
A similar issue arises even for PCR tests that are applied to more conveniently located samples. It has been documented that when samples are taken from just inside the nose or from snot or saliva, they often do not register RNA remnants even if a deeper sample would find some. However, one possibility is that this is because viral remnants only appear and remain in those places during the time when the viral load is at its peak and the patient is most infectious.
Thus, it is possible that you want to take a test from snot or saliva (which also has the advantage of being easier to retrieve) and for a negative result to be if those are clear based on the same threshold you use for a PCR test taken with a deeper nasal swab. Technically, this test would have lower sensitivity based on usual calculations of sensitivity but, in actuality, conditional on the context (that is where the sample is taken from) it is actually more sensitive for the thing you are interested in. In other words, you may get a clearer signal from a different method of taking samples for the purpose of clearance. At present, requirements for sensitivity and specificity do not take this into account and so are conditioned on searching for small viral loads pooled with higher ones.
Indeed, labs are not allowed (!) to report Ct results at all which means that key information is being thrown away let alone being to track how the viral load is changing for a patient. Surely, it should always be reported and, moreover, encourage a future test with another Ct value to establish where someone is in the cycle. People are potentially being asked to quarantine or being contact traced erroneously.
The Clearance Issue
If you are running a dentist office, hospital or airline, what you want is a test that clears an individual as safe for a certain period of time. Because PCR tests can take a day or more to yield results, for clearance, organisations have been looking at rapid point of care tests that can return a result in a few minutes. One such test is the Abbott ID Now system. It is a PCR test and machine that was developed way back in March. It has been controversial.
The issue is sensitivity. An initial study comparing it to gold standard PCR tests (actually even more sensitive than the ones commonly used) found that its sensitivity was low; 87% rather than 98% for the gold standard. This is a problem because, as noted earlier, for clearance you want tests to have high sensitivity implying that there are fewer false negatives or people who are infected that you clear.
The problem with this scoring is that it is not calibrated for the decision context which is for infectiousness rather than infection. You do not care if people are infected but not infectious for clearance. The initial NYU study was published in August and noted that ID Now failed when there were low viral loads but omitted the data on that issue. The pre-print has that data which makes it clear that ID Now was highly sensitive for infectiousness.
In other words, for clearance, sensitivity for rapid tests may well be higher enough conditional on that decision context and sensitivity scoring based on diagnosis will be misleading. Suffice it to say, there is a regulatory discord here that will have a meaningful impact on businesses hoping to rely on clearance testing.
Match the Test to the Decision
The bottom line is that tests need to be scored and evaluated for the decision context they are being used for. Our regulatory processes are all designed for diagnosis and this is a major discord in solving the pandemic information gap with testing. In tomorrow’s newsletter, I will examine what this means for our hopes of using testing as a virus mitigation strategy.