Test Week: The Information Gap
How tests plug the pandemic information gap depends on the type of decisions they are used to inform.
Welcome to Plugging the Gap (my email newsletter mainly about Covid-19 and its economics). My goal is for several posts a week explaining economic research and the economic approach to understanding the pandemic. (In case you don’t know me, I’m an economist and professor at the University of Toronto. I have written lots of books including most recently on Covid-19. You can follow me on twitter (@joshgans) or subscribe to this email newsletter here).
This week, I am looking at testing for the presence of the coronavirus in individuals. Various aspects will be developed in the coming days. For today, I will argue that:
The value of testing is that it solves an information problem for pandemics and, thus, can potentially save on other pandemic control costs that tend to be ‘always on’ solutions that impact on a population of individuals rather than just on the infected.
The particular type of tests you want depends on the type of decision you are making and there are four types of decision: diagnosis, clearance, surveillance and mitigation.
A key variable that impacts on how informative tests are is the prevalence of the virus in the population at any given time. If prevalence is too low, tests are noisier signals and less useful.
Other information gathering activities such as tracing can complement testing in solving pandemic information problems especially when testing is costly.
The second and longer edition of my book is entitled The Pandemic Information Gap: The Brutal Economics of COVID-19. This is also the Gap referred to in this newsletter’s name. The reason for that title is that book, unlike the first edition, doubles down on the idea that pandemics and their control is fundamentally an information problem.
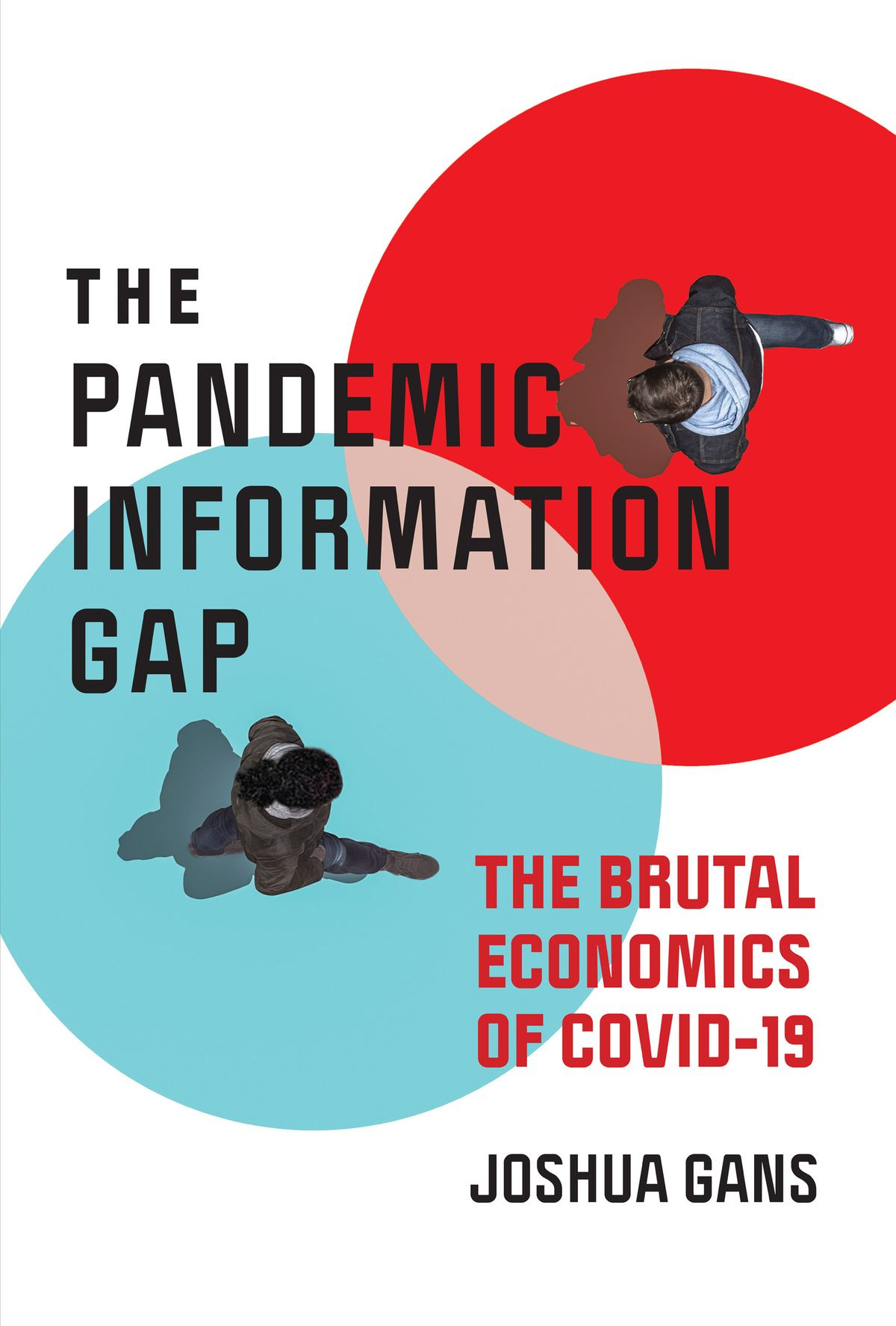
The logic is very simple. Pandemics, like Covid-19, are a problem because they spread from infectious people to susceptible people. If we think of pandemics solely as a health problem we focus on protecting people’s health by developing a vaccine or by isolating people from one another. These options are either slow or very costly. By contrast, if we think of pandemics as an information problem what we focus on is identifying infectious people and isolating them, and only them, from others. Solving this problem requires a method of identifying infectious people.
For some pandemics, the virus/disease solves the information problem for us by having people show symptoms when they are infected. This is what happened for SARS, MERS and Ebola and consequently, those pandemics were suppressed in a matter of months. For Covid-19, countries like Taiwan, Iceland, Mongolia and South Korea treated Covid-19 as an information problem from the start. They quickly developed tests and used them to test broad sections of the population and alongside this used contact tracing to help them identify who was more likely to be infectious. For Covid-19, which was a disease where people were infectious without being symptomatic, these countries turned Covid-19 into SARS.
None of this is news but, yet, many countries have not taken seriously the idea that Covid-19 requires solving the information problem. To be sure, testing has improved and there are many countries who have a test, trace and isolate regime that has done great work in suppressing outbreaks and are now assisting in avoiding larger lockdowns. Where outbreaks have emerged following suppression — e.g., in Australia and New Zealand — it is not because the information problem was treated lightly but that there were leaks in the ‘isolate’ action that needs to be taken and enforced. Covid-19 is a sly beast and can quickly run rampant when a population is going about their business as normal. There will be no perfect solution but the evidence suggests that you can avoid the costs associated with trying to solve Covid-19 as a health problem by prioritising solutions to the information problem.
Given this, I am going to devote this entire book to testing and where we are on getting to a testing regime that can operate at a scale where we can avoid all of the costs associated with the day-to-day dealing with Covid-19. In a blog post, economist John Cochrane neatly summarised this goal:
A vaccine is a technological device that, combined with an effective policy and public-health bureaucracy for its distribution, allows us to stop the spread of a virus. But we have such a thing already. Tests are a technological device that, combined with an effective policy and public-health bureaucracy for its distribution, allows us to stop the spread of a virus.
When do tests have value?
At a broad level, tests have value because they can lead to better decisions to reduce the spread of the virus throughout the population. At the level of an individual place, their value arises because of the costs associated with what you have to do in their absence — which is to treat every individual who comes into your place as equally likely to be infected and cause an outbreak amongst others.
A couple of months back, myself along with Ajay Agrawal, Avi Goldfarb and Mara Lederman explained, in an article published in the MIT Technology Review, that when everyone is equally risky, managers have to invest in ‘always-on’ solutions. Here was our description of these:
All sorts of decisions that previously would have been made on the basis of productivity and efficiency now need to also consider the possibility of infection. In the restaurant industry, the flow of people in and out of the kitchen is now an infection-risk management problem. In the retail fashion industry, decisions about whether to open changing rooms or allow customers to try items on are now infection-risk management problems. Moving from physical to digital documents now reduces infection risk as well increasing efficiency and wasting less paper. The risk of transferring the virus by exchanging cash increases the relative benefits of digital payment systems.
To date, we have seen two broad types of always-on solutions. The first kind do not change the number or nature of interactions but aim to make those interactions less risky. Things like masks, hand sanitizer stations, and plexiglass screens at reception desks and store checkouts all fall into this category.
The second kind are solutions that aim to make people interact less. These include redesigned physical spaces (to minimize interactions or high-touch surfaces), redesigned workflows (to enable work to be done in parallel or sequence rather than jointly), and redesigned people-management processes (to minimize interactions across groups or teams). Reductions in capacity—whether of employees (through layoffs and furloughs) or customers (through limits on occupancy)—fall into this category as well.
Always-on solutions impose additional costs on business. There are direct costs for things like protective equipment and more frequent cleaning. If the always-on solution involves reduced capacity, profits will fall. Finally, reengineered spaces, workflows, and processes may lead to lower productivity, greater inefficiency, or unhappier workers. Of course, certain changes could increase productivity. Some businesses, especially those in congested cities like New York, report that work from home has made them more productive, mainly because it eliminates long commutes.
Different types of businesses lend themselves differently to always-on solutions. It’s easier to maintain social distancing in garden centers than in hair salons. Some businesses are choosing not to open even if they are allowed to: many restaurants have elected to keep their dine-in services closed because with social distancing, they can’t allow in enough customers at a time to offset the costs of cleaners and wait staff.
Depending on your business, the costs to you of having an always-on solution can be different. When those costs are high, that is when there is greater value to finding a way to identify those who are more likely to be infected and using that information to protect others rather than treated everyone as equally dangerous. Schools are one of the places where these costs have become significant. Toronto has allocated $100 million for these types of solutions so that it can safely reopen schools next month. Suffice it to say, even with these costs, the education experience will not be anywhere near what would normally be provided. Thus, saving costs is not just about dollar expenditures but about what can be achieved as a pandemic hangs over our heads.
What are tests for?
Unlike what many students believe, tests aren’t conducted just for the fun of it. A test is a means of gathering the information you need to make a better decision. If you don’t intend to make any decisions, there is no need to undertake any testing. The point of saying this rather obvious statement is that before advocating or developing a test you want to clearly articulate the decision you are trying to make.
With regard to testing for the presence of a coronavirus like SARS-CoV2, there are four broad decisions for which a test could improve outcomes. These are based on categories identified by Carl Bergstrom.
Individual diagnosis: Whether to treat or monitor a particular patient for coronavirus complications.
Clearance: Whether to allow someone to interact with others or not in a close physical setting such as treating or being treated as a patient by a doctor, dentist or other health professional or being able to travel.
Surveillance: Whether to engage in non-health interventions to prevent the spread of disease.
Mitigation: Whether to isolate a person or not from other people.
For each of these decisions, knowing whether someone is infected or not is valuable information in that you would take different actions depending on that information. Other than that they are very different decisions involving different actions and different consequences. As we will see later in the week, these decisions also differ in the usefulness of whether tests can be cheap and widespread, whether the tests have to be accurate in one direction or another, and whether test results can be received quickly or not.
Prevalence changes what we can learn from tests
As we will discuss in a future newsletter, tests are imperfect. They result in false positives (people who test as infected but are not) and false negatives (people who are infected but the test clears). Different types of decisions change how you feel about such errors. But in all cases, when there are too few people around who are actually infected, the less clear the signal is from any test.
Let’s suppose for the moment, that if a test is performed, it will identify whether someone is not infected when they are actually not infected, 95% of the time. That means that 5% of the time the tests will come back positive but the tested individual is not infected. For instance, I’m pretty sure I am not infected at the moment. So if I took that test there is a 5% chance that it will be positive and I will be sent to isolation. Given my beliefs, that makes the test pretty risky to take!
My beliefs aren’t simply a result of my unfailing optimism and the fact that I have steered clear of people (although that is also information as we will see in a bit). It is a result of the public information regarding the low rates of infection currently here in Toronto. Even at the height of the outbreak here, there was a 1% chance a random person had the virus. Thus, we could all be 99% sure we didn’t have it.
The problem isn’t that fact, nor the fact that the test is imperfect. Instead, it is how we use tests results. We use them as if they are perfect. Negative you are free. Positive you are imprisoned. In reality, what the test is doing is causing our assessment of the probability that the test subject is infected or not to change.
So let’s look at those probabilities. To get into that, we need, first to ask, what is the chance that a test of any random person is likely to return a positive result. Let’s suppose that if you are infected, you will test positive and not negative. This means that if any random person is positive, which has a 1% chance of being true, they will test positive with probability 0.01 x 1. On the other hand, if a random person is actually not infected, which has a 99% chance of being true, they will test positive with probability 0.99 x 0.05 or 0.0495. Thus, if you test a random person, you can expect that the test will show a positive result with 1% + 4.95% or 5.95% probability.
Now, given this, what is the probability that I, who have just received a positive test result, am actually infected? Well, we expect that a share 1/5.95 of all positive tests will be from infected people and a share 4.95/5.95 will be from negative people. Thus, the probability that I am actually infected given that I have just received a positive test result is approximately 17% (= (1/5.95) x 100). Clearly, you can reasonably think that I am more likely to be infected as a result of having this test, but there is also a really high chance that I am not infected. So do you isolate me or not? It is a harder question as on these odds it is still far more likely that I am not infected. The point, however, is that when prevalence is low, treating a positive test result as if I am positive for sure is incorrect reasoning.
All of this was just a simple application of Bayes Theorem but without actually forcing the innards of that on you. But if you want that, this article is a good place to start. Also, as I will discuss in a later newsletter, for many of our coronavirus tests, false negatives rather than false positives is the issue but even in those cases, the reasoning I have applied here carries over.
Contact tracing helps tests solve the information problem
The difficulty in interpreting test results changes if we know more about the person being tested. This can come from contact tracing. When someone has been found to have Covid-19, in many countries, public health officials trace people that person has been in contact with over the previous week or so. The reason this is done is because it may take some days before an infected person is verified as such. With contact tracing, individuals identified as having contact with an infected person are more likely to have caught the virus.
What is the new probability that you are infected given that you have had close contact with an infected person? It won’t surprise you that the date on this is scant but this one study I found cited rates of between 11% and 16%. So let’s assume 15% for the sake of argument and bear in mind that these figures are illustrative and not to be used for actual anything.
Now the scenario is that I have been found by contact tracers and had contact with an infected person so the probability, absent a test that I am infected is now 15%. With this new information, I am tested and test positive. So what is the actual probability that I am positive? Using the same method as before, note that a random person flagged to be exposed by an infected person has a 15% + 4.25% (= (0.05 x 85)100) = 19.25% chance of testing positive. So if I test positive, there is an almost 78% (= (15/19.25) x 100)) probability that I am actually infected. This certainly makes a big difference.
What this demonstrates is that the informativeness of tests that are imperfect can be greatly enhanced if you have some information that causes you to believe a person is more likely to be infected. Thus, contact tracing goes hand in hand with testing even if you weren’t worried about allocating scarce testing resources properly.
Forecast
There will be more posts on testing throughout the week. Just to preview, I’ll do a deeper dive on what imperfect test results mean for different decisions tomorrow. After that will be a focus on methods to drive down the cost and increase the speed of testing.