Looking for Covid-19 in other places
Welcome to Plugging the Gap (my email newsletter about Covid-19 and its economics). In case you don’t know me, I’m an economist and professor at the University of Toronto. I have written lots of books including, most recently, on Covid-19. You can follow me on Twitter (@joshgans) or subscribe to this email newsletter here.
I thought it was time to revisit another way of looking for Covid-19 outbreaks. Early on in the pandemic, I looked at Google Trends and found that you could see signs of the pandemic from google searches such as “loss of smell.” Here is the most recent trend for the US (where Anosmia is the general topic for these types of searches).
You’ll notice that it peaked in March (around the time news of this symptom became public) although there was a little rise before. But it is rising again. This is interesting but also noisy. Drill down to subregions and the data is harder to parse.
Anyhow, the other day I was alerted to the following tweetstorm by, economist, Craig Palsson. He had come across another person, Kate Petrova, who inspired by another tweet delved into Amazon reviews of scented candles; in particular, those complaining that the scented candles they received were, in fact, unscented. That makes for interesting reading.

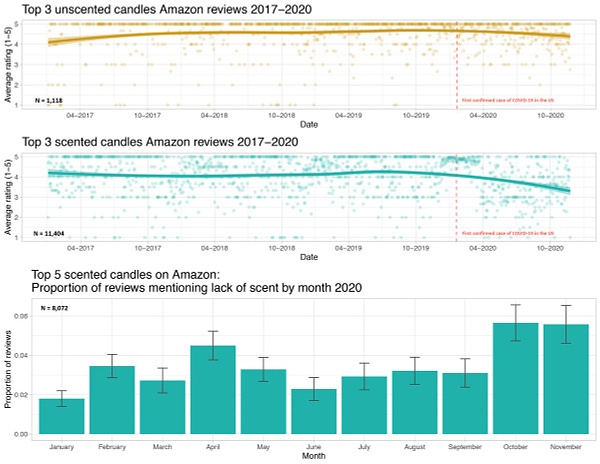

This is the most interesting graph.

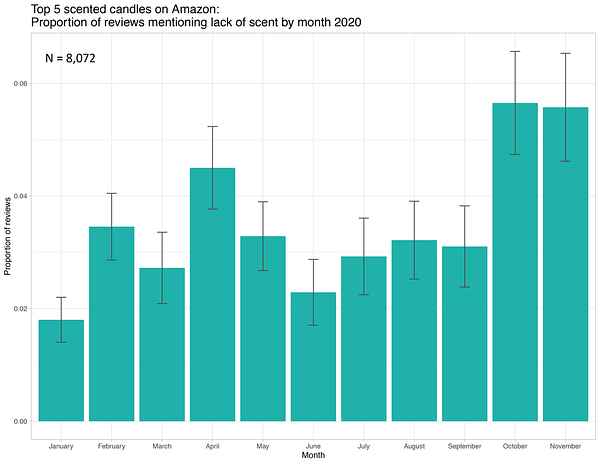
Now, this all might not be perfect. There is a certain type of person who buys a candle and, most likely, there are seasonal elements. But it is an interesting potential window on the pandemic. What it shows is that we have many more tools for pandemic surveillance and there might be fruitful ways of having AI work through a ton of data to find other indicators. To be sure, this is less useful for Covid-19 than it may be to get early warning of trends for the next pandemic.
Some of this work is already being done. A recent paper by Cornelia Ilin, Sébastien E. Annan-Phan, Xiao Hui Tai, Shikhar Mehra, Solomon M. Hsiang, and Joshua E. Blumenstock takes a corpus of mobility data from Google, Facebook, Baidu and SafeGraph. With this data, the researchers were able to generate a more accurate short-term forecast of Covid-19 cases than epidemiological approaches alone. This is because mobility can cause infections (although it can also go the other way).
This type of analysis will likely be useful for the current pandemic. The point here is that in today’s world there is an opportunity to greatly build up surveillance of public health-related information. Some years ago, Google thought they could do this for the flu. It turned out their predictive algorithm wasn’t stable. But the idea was solid. We just need to continue to push to find the right approach.