Data Week: How to collect data
Welcome to Plugging the Gap (my email newsletter about Covid-19 and its economics). In case you don’t know me, I’m an economist and professor at the University of Toronto. I have written lots of books including, most recently, on Covid-19. You can follow me on twitter (@joshgans) or subscribe to this email newsletter here.
This week is data week at this newsletter. Today’s newsletter starts and pretty much ends up in the sewer. Regular readers will recall that SARS-CoV-2 RNA remnants have been detectable in sewerage sampled at treatment plants. I argued that, while this might help detect outbreaks at a local population level, it wouldn’t necessarily inform targetted interventions. A new paper co-authored by Richard Larson, Mehdi Nourinejad and my Rotman colleague, Oded Berman shows that a more finely grained exploration is possible.
Their innovation is twofold. First, they collect data at a manhole level. That is easier said than done as there are lots of manholes and they are all interconnected. This brings their second innovation: algorithms to sort through the potential mess.
Our data source is wastewater sampled and real-time tested from selected manholes. Our algorithms dynamically and adaptively develop a sequence of manholes to sample and test. The algorithms are often finished after 5 to 10 manhole samples, meaning that—in the field—the procedure can be carried out within one day. The goal is to provide timely information that will support faster more productive human testing for viral infection and thus reduce community disease spread.
Put simply, by collecting data closer to the (ahem) source, information can be generated more rapidly than waiting for it to flow downstream to treatment plants. This figure illustrates the issues.
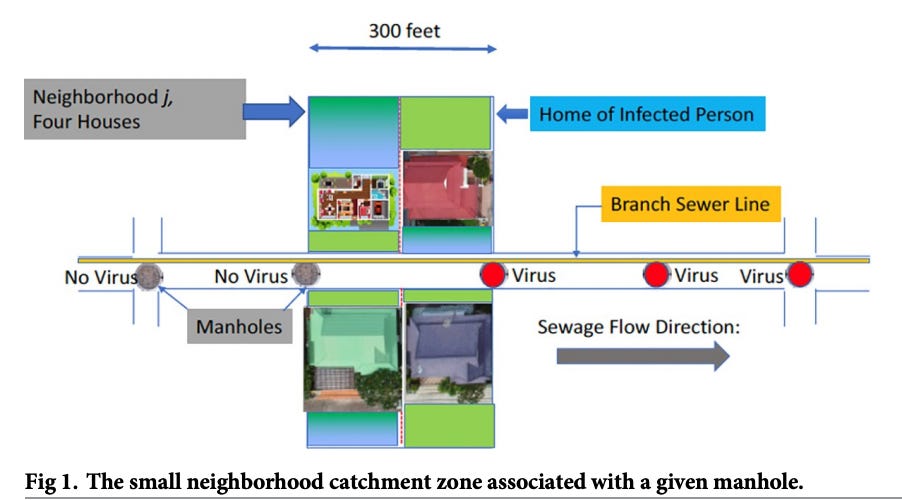
They write:
Any test of the upstream manhole, if it exists, would reveal no COVID-19 from the residence of the infected person(s). But the closest downstream manhole would provide that evidence. We seek to find that closest downstream manhole. If successful, we can then reduce our search for the infected person(s) to residences of only a few houses (i.e., all those houses first inputting to the same downstream manhole). By such targeted human testing, we may be able to stop any spread from Patient(s) Zero to the rest of the community.
This is quite a challenging process:
Leveraging the tree graph structure of the sewage system, we develop two algorithms, the first designed for a community that is certified at a given time to have zero infections and the second for a community known to have many infections. For the first, we assume that wastewater at the WTP has just revealed traces of SARS-CoV-2, indicating existence of a “Patient Zero” in the community. This first algorithm identifies the city block in which the infected person resides. For the second, we home in on a most infected neighborhood of the community, where a neighborhood is usually several city blocks
It is certainly fascinating and shows the potential of using sophisticated statistical techniques to provide a deeper understanding of outbreaks.
Not surprisingly, not only do you need a solid sampling process for manholes, you also need good mapping data on the nature of the system itself. They show how this can be done for a small New England (where else?) town. “Top panel depicts a map of the Marlborough, Massachusetts wastewater removal system; the red arrows represent the direction of flow. The green circles are the manholes of the sewage pipe system. Bottom panel is a reduced network depiction of Marlborough’s sewage network. “WTP” in both panels represents the wastewater treatment plant”
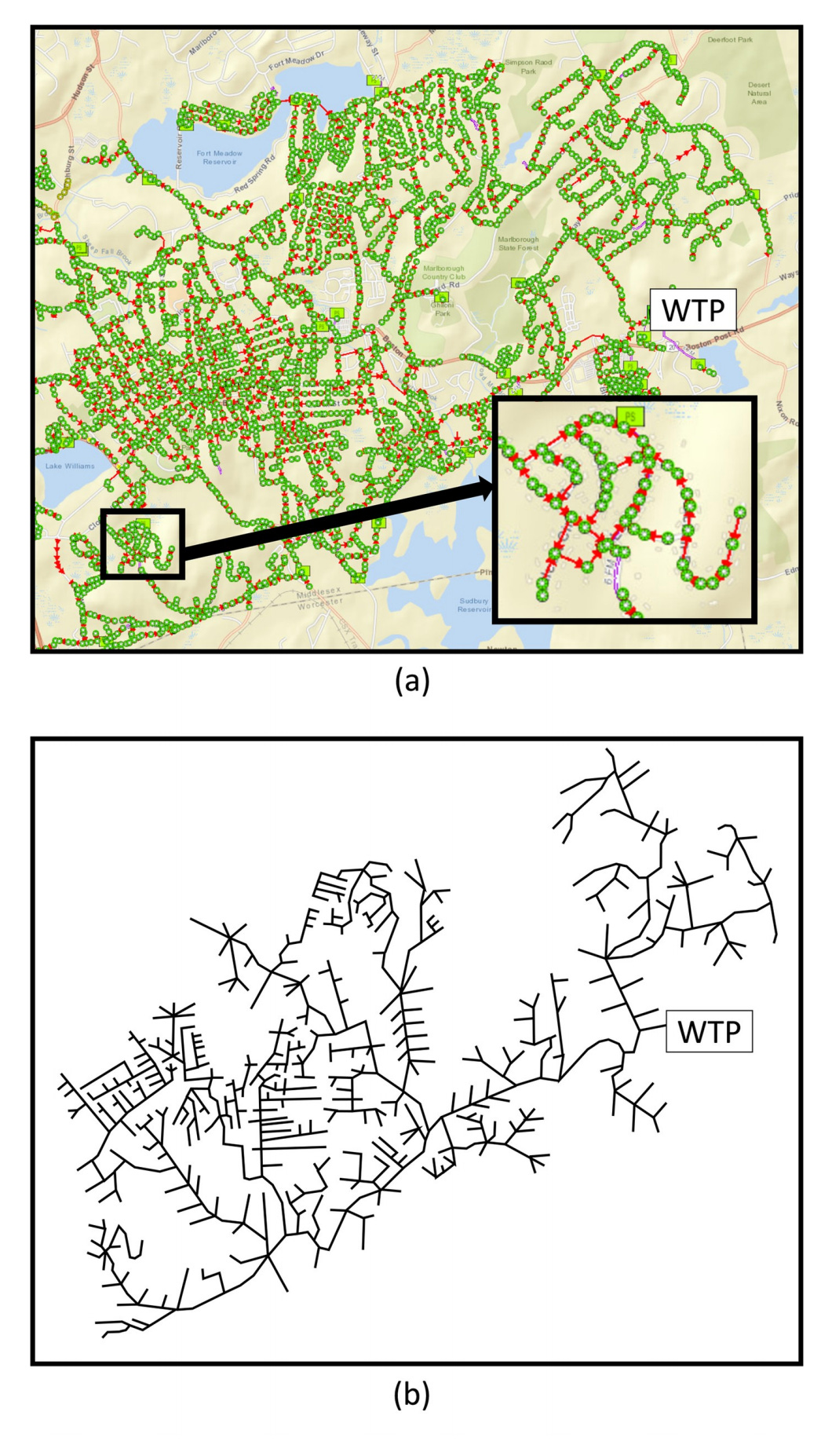
In the end, using a Bayesian framework, the researchers show how to convert sewage flows into “probability flows” to assess the right place to collect samples. Suffice it to say, if you are in the business of collecting stool, you want to optimise anything you can. With their algorithm, you can find a hot spot neighbourhood in this town with as few as 4 samples. Finding patient zero in a new outbreak is a little harder but 10 samples will do the job.
That said, it does presume relatively stable depositing behaviour by people in the town. The authors recognise this but have yet to work out how to deal with it.
We did not devote attention to evaluating the relative Bayesian probabilities. Their careful estimation for this procedure could be an entirely separate paper, and in practice, could result in much-improved performance. For instance, a neighborhood in which the majority of people have jobs that require leaving the house and working in an environment with substantial human interaction is likely to generate more COVID-19 cases than one in which most residents can work from home via the Internet. These differences can be expressed by markedly different values of the Bayesian probabilities assigned to neighborhoods.
I couldn’t have put it better myself.
Suffice it to say, if anyone deserves a Sewage Treatment Plant named after them, it is these intrepid Covid sewage researchers.